There has been some interest in our recent preprint describing Oxford Nanopore MinIONTM sequencing for 16S rRNA microbiome characterization and I was asked to write a post for microbenet on this technology. Disclaimers – this paper is a work in progress – our paper has not yet been peer-reviewed and we are continuing to revise our work and conduct additional experiments based on feedback. We welcome feedback as we continue to work on this topic. I was also part of the Oxford Nanopore ‘Early Access Program’ and was able to access the platform before release at a discounted cost. It is worth noting others are working on this topic as well: Benítez-Páez et al., Shin et al., and Mitsuhashi et al.
The MinION platform (pictured below) is a portable DNA sequencing platform that is capable of providing long read sequence data in near real time. Additional information is on the Oxford Nanopore website: https://nanoporetech.com/ and many academic reviews exist for specific applications. The flowcell can be washed after each run and reused multiple times with decreasing sequence output. Currently, a flowcell is ~$900 and can be used at least 6 times for relatively short runs. The primary drawbacks of this technology are a relatively high error rate (~8% per-base error rate) and higher per-base costs than other current technologies (e.g. Illumina).
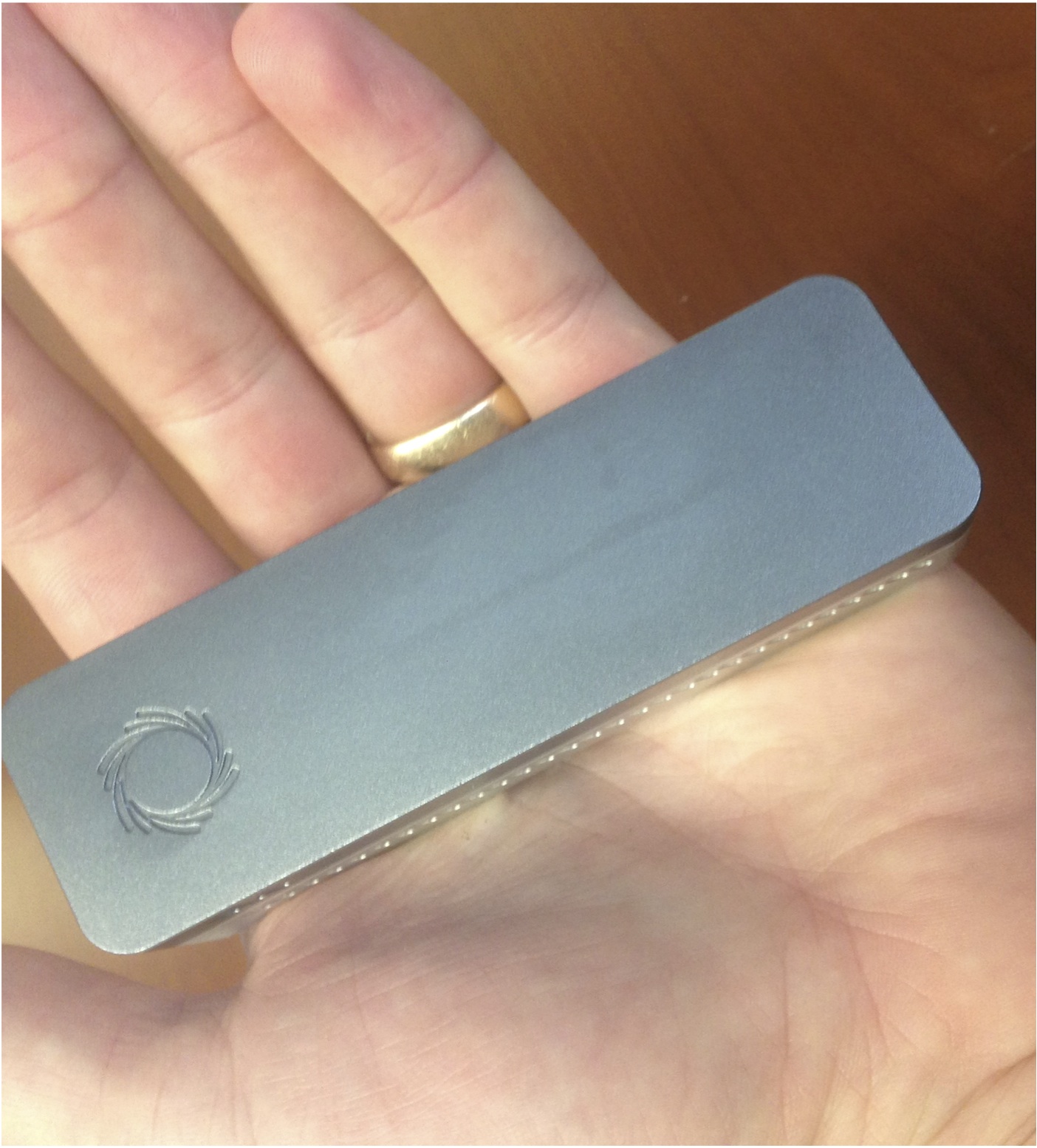
The appeal of using MinION for 16S rRNA sequencing is the portability, the potential to get near full-length 16S rRNA reads, and the ability for rapid (same day) sequence data. The capital costs are also low (a laptop), which is a step forward in the ‘democratization of sequencing’. While there are many potential applications, some may include sample screening prior to sequencing on another platform, sequencing in the field, or sequencing in the clinic for patient monitoring. The obvious challenge is the error rate.
To initially evaluate the potential of this technology, we sequenced 16S rRNA sequences from pure-culture E. coli and P. fluorescens, as well as a low-diversity sample from hydraulic fracturing produced water that we had previously analyzed using Illumina sequencing. We actually evaluated many more samples, but were forced to exclude them due to sample carryover between washes, which I discuss below.
We attempted to cluster the pure-culture reads into Operational Taxonomic Units (OTUs) but all approaches we tried failed – using a de novo approach at the typically used 97% similarity level, >99% of reads clustered into unique OTUs. Taxonomic assignment using the Ribosomal Database Project’s Naïve Bayesian Classifier was more successful, achieving 93.8% and 82.0% annotation accurate at the phyla and genus levels, respectively (shown below). It will be necessary to examine more diverse pure-culture samples to determine the role of database representation on annotation accuracy. Comparison of the mixed community sample had much higher similarity when using ‘weighted’ (abundance) measures than when using ‘unweighted’ (presence/absence) measures. Taken together, these results suggest that this approach is potentially useful for initial assessment of microbial communities and for observing broad microbial community shifts. At this stage, this approach has limited utility for ‘fine-grained’ microbial community resolution.
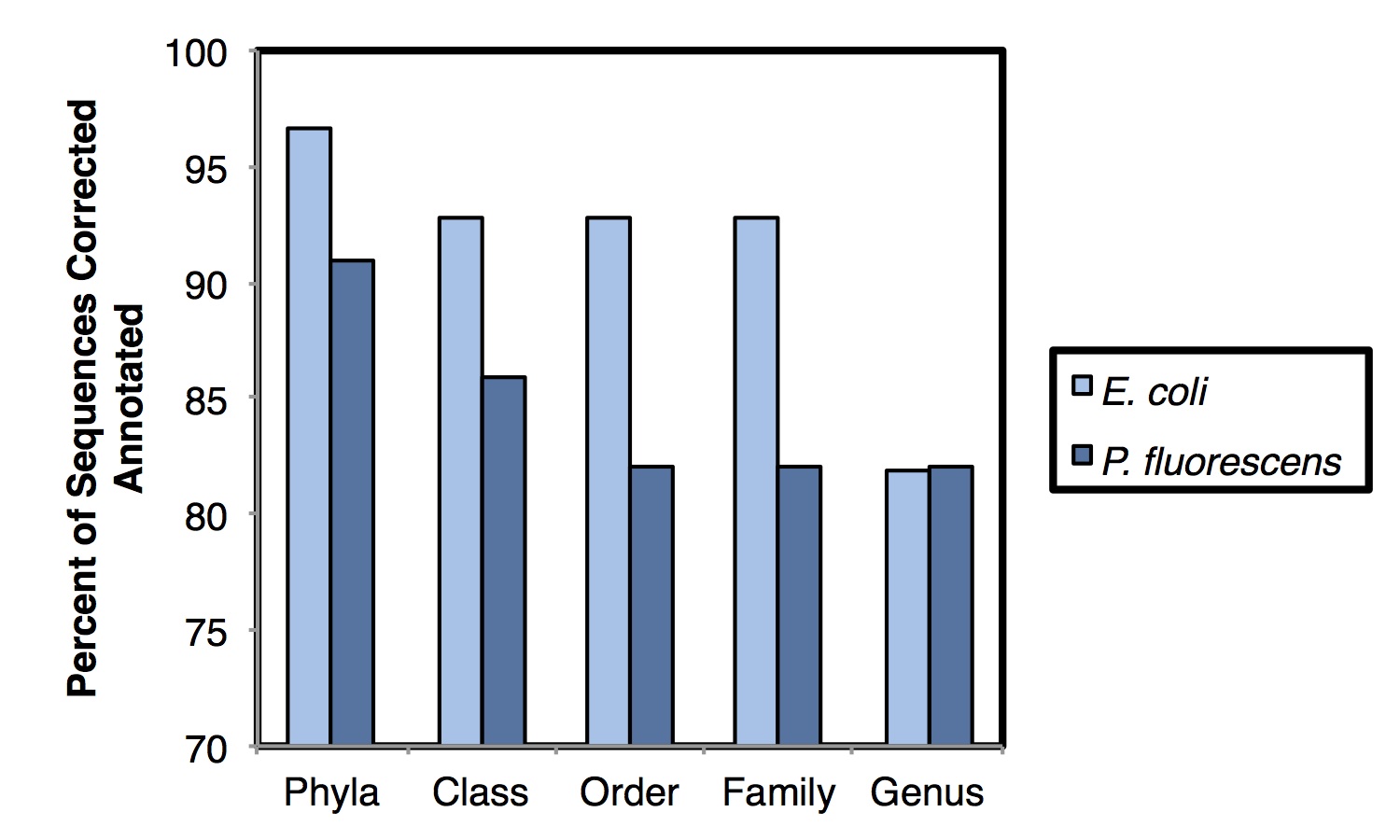
When analyzing pure-culture sequence data we also observed apparent between-wash carryover of ~10% of sequence reads. We have since seen some notes to this effect in the literature and a technical note by Oxford Nanopore. Those working on this technology should be aware of this phenomena (especially if working with mixed-culture samples where the carryover would be less obvious). Improved between-run washing or sample barcoding should help to alleviate this challenge.
Dr. Kyle Bibby is an Assistant Professor in Civil and Environmental Engineering at the University of Pittsburgh. You can find him on twitter @kylejbibby
Edit 1/30/17 to fix typo on weighted vs. unweighted measures.
RE OTUs. With a 10% error rate, assuming random errors, two identical rRNA genes could differ by up to 20% on the MinION platform. So no surprise clustering at 97% didn’t work. What would be really interesting is to see some sort of sequence alignment dendrogram/tree so we can predict at what point OTUs actually exist. Alternatively we could simulate a community and then simulate reads with 10% error and see where the OTU cut-off should be.
Great point – we did this analysis for OTU similarity threshold vs. percentage of sequences in unique OTUs. I will try to figure out how to link here.
Update: https://twitter.com/kylejbibby/status/826148100762918912
Is it possible to circularize amplicons to increase read accuracy?
This is somewhat what they are trying to do with the new chemistry that just reads both sides of the DNA molecule to develop a consensus read (i.e. consensus of 2 reads), but I do not think it would be possible to circularize it as you would using Pacbio chemistry.